Transforming Fraud Detection and Software Management in Real-Time
Can traditional fraud detection methods keep pace with the sophistication of cyber threats? As organizations grapple with increasingly complex challenges, the answer is a resounding no. The landscape of digital security is evolving, and with it, the necessity for robust, AI-driven solutions that provide real-time fraud detection has never been more critical.
A Deep Dive into Real-Time Software Management and its Implications
When every second counts, being able to swiftly identify and respond to fraudulent activities is vital for safeguarding assets and maintaining operational integrity. This article explores how machine learning algorithms are transforming user behavior analysis and enhancing the ability to detect anomalies effectively.
We will navigate through the innovative technologies at play, examine case studies that illustrate their impact, and discuss the future of fraud detection in an era dominated by cyber threats. By the end of this article, readers will gain valuable insights into the intersection of AI technology and fraud prevention, equipping them with the knowledge to adapt to this changing landscape.
Understanding the Fundamentals of AI-Driven Fraud Detection
AI-driven fraud detection utilizes advanced algorithms and data analytics to identify and prevent fraudulent activities in real-time. This technology leverages machine learning and artificial intelligence to analyze patterns in user behavior, helping organizations catch irregularities before they escalate into significant threats.
The key principle behind AI-driven fraud detection is its ability to learn from vast amounts of data, refining its models to improve accuracy over time. Unlike traditional methods, which often rely on static rules and thresholds, AI systems adapt to new behaviors and emerging threats, making them more effective in dynamic environments.
For example, consider an e-commerce platform that employs AI to monitor transactions. By analyzing historical data, the AI can establish a baseline of normal user behavior. When a transaction deviates from this pattern—such as a sudden purchase from a new location—it triggers an alert for further investigation, allowing the company to act swiftly to protect its assets.
In essence, AI-driven fraud detection transforms the approach to cybersecurity by enabling proactive measures rather than reactive responses. By harnessing the power of AI, organizations can not only mitigate risks but also enhance their overall operational integrity and customer trust.
The Current Landscape of AI-Driven Solutions in Fraud Detection
The integration of artificial intelligence into fraud detection and software management is reshaping the cybersecurity landscape. Organizations are increasingly adopting AI technologies to improve their defenses against sophisticated cyber threats, which have grown exponentially in frequency and complexity. According to a recent report, the global AI in the cybersecurity market is projected to reach $38.2 billion by 2026, reflecting a significant growth potential as companies recognize the value of AI-driven insights.
Key players in this space include established technology giants such as IBM, Microsoft, and Palantir, alongside innovative startups focused on niche solutions. These entities are leveraging machine learning and advanced analytics to enhance their fraud detection capabilities. Trends such as the rise of behavioral analytics and real-time risk assessment are becoming standard practices, enabling organizations to respond to potential threats with unprecedented speed.
Recent developments in AI technology have led to breakthroughs in anomaly detection, allowing systems to learn from historical data and identify unusual patterns that may indicate fraudulent activity. Furthermore, regulatory changes are pushing organizations to adopt more robust fraud detection systems, creating a challenging yet opportunistic environment for AI applications.
As organizations continue to navigate this dynamic landscape, the importance of adopting AI-driven approaches for fraud detection cannot be overstated. These advancements not only help mitigate risks but also enhance operational efficiency, making them essential tools for safeguarding assets in today's digital era.
The Importance of AI in Fraud Detection and Software Management
The integration of AI technologies in fraud detection and software management is not merely a trend; it is a transformative force reshaping how organizations operate in a digital landscape fraught with risks. The significance of leveraging AI lies in its ability to analyze vast amounts of data in real time, which enhances the speed and accuracy of fraud detection processes. By employing machine learning algorithms, businesses can uncover hidden patterns in user behavior that indicate potential fraudulent activities, significantly mitigating financial losses and reputational damage.
Applications of AI extend beyond fraud detection into various domains such as transaction monitoring, risk assessment, and compliance management. For example, financial institutions harness AI to scrutinize transactions in real time, swiftly flagging suspicious activities that warrant further investigation. Similarly, e-commerce platforms utilize AI-driven solutions to prevent chargebacks and identify fraudulent returns, thereby preserving revenue and customer trust
Looking ahead, the future potential of AI in this field is vast. As algorithms become more sophisticated and data sources diversify, the ability to predict and prevent fraud will improve dramatically. Organizations that embrace these advancements will not only enhance their security posture but also create a competitive edge by fostering a culture of proactive risk management.
Moreover, the relationship between AI and other technological advancements, such as blockchain and cybersecurity measures, is becoming increasingly vital. Integrating AI with these concepts can enhance overall system resilience, enabling organizations to respond effectively to evolving cyber threats. This synergy emphasizes the need for businesses to adopt a holistic approach to technology implementation, recognizing that AI is a cornerstone of modern security strategies.
Trends Shaping the Future of AI in Fraud Detection
The landscape of fraud detection is undergoing a significant transformation, driven by innovative trends that leverage artificial intelligence. One prominent trend is the integration of advanced machine learning algorithms, which are redefining how organizations approach fraud prevention. These algorithms enhance predictive capabilities by continuously learning from vast datasets, allowing companies to stay one step ahead of emerging threats.
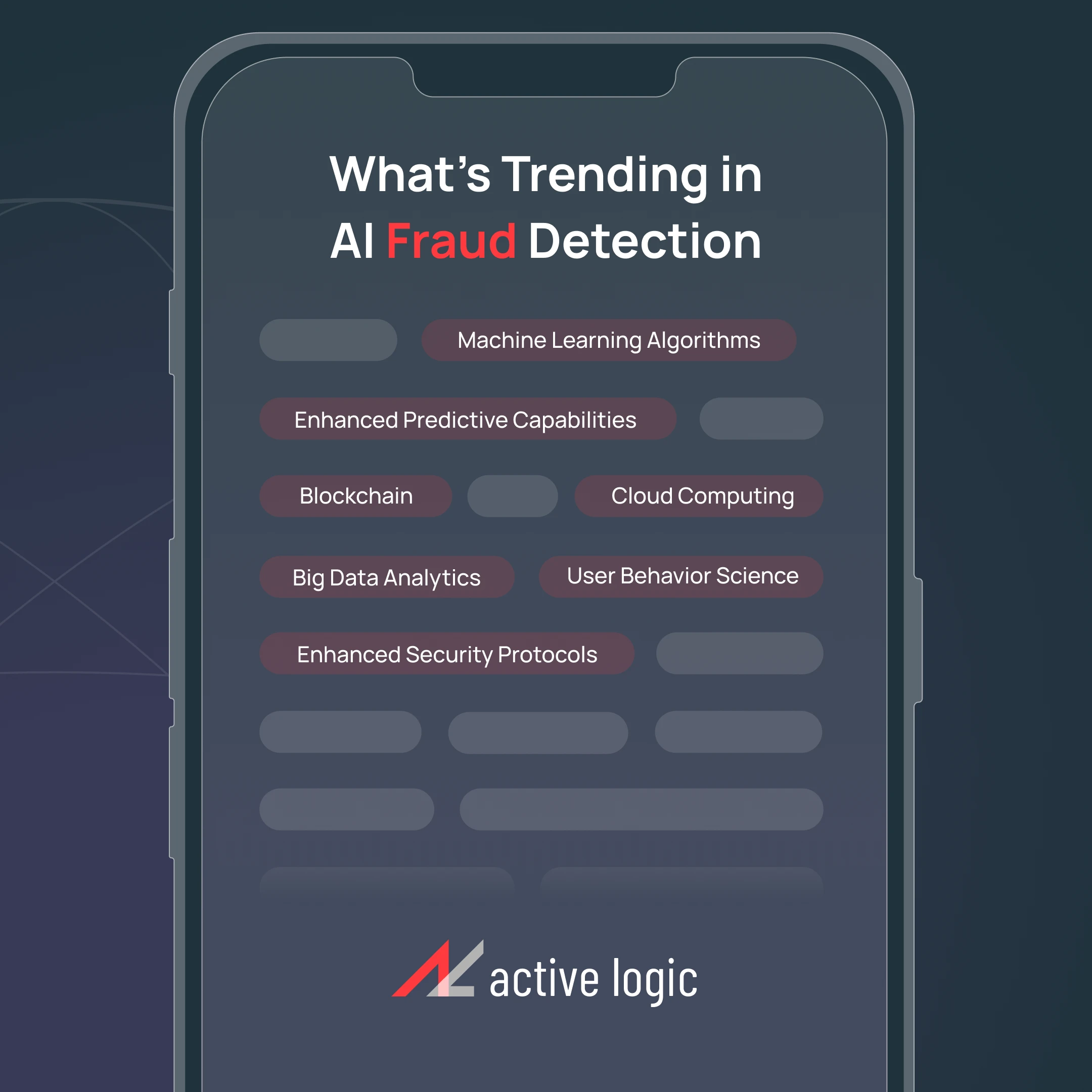
Trends Shaping AI Fraud Detection Efforts
Technological advancements, particularly in cloud computing and big data analytics, are further bolstering these AI-driven solutions. These technologies enable the analysis of extensive user behavior patterns in real-time, facilitating the swift identification of anomalies that may indicate fraudulent activity. As a result, organizations can implement proactive measures to mitigate risks effectively.
Looking ahead, the potential for AI in fraud detection is immense. Future developments may include the incorporation of deep learning techniques that not only improve detection accuracy but also reduce false positives, which have historically plagued the industry. Additionally, the use of AI in conjunction with blockchain technology could lead to enhanced security protocols that further safeguard organizational assets.
Real-world case studies illustrate the impact of these trends. For example, major financial institutions have adopted AI-driven platforms that analyze transaction data in real-time, resulting in a marked decrease in fraudulent transactions. These advancements not only protect assets but also enhance operational integrity, demonstrating the critical role of AI in reshaping fraud detection strategies.
AI-Driven Fraud Detection vs. Traditional Methods vs. Rule-Based Systems
The landscape of fraud detection is ever-evolving, with organizations exploring various methodologies to combat rising cyber threats. This section provides a comparative analysis of three prominent approaches: AI-driven fraud detection, traditional methods, and rule-based systems.
Alternatives Identified
-
AI-Driven Fraud Detection:
Utilizes machine learning algorithms to analyze patterns and detect anomalies in user behavior in real-time.
-
Traditional Methods:
Often rely on manual processes and human oversight to identify fraudulent activities, typically involving more reactive measures.
-
Rule-Based Systems:
Operate on predefined rules and thresholds to flag potentially fraudulent transactions, which can be rigid and less adaptive to new threats.
Comparison Table
Feature | AI-Driven Fraud Detection | Traditional Methods | Rule-Based Systems |
---|---|---|---|
Speed | Real-time analysis | Slower response time | Moderate responsiveness |
Adaptability | Highly adaptive; learns over time | Limited adaptability | Rigid; requires updates for new threats |
False Positives | Lower false positive rate | Higher false positive rate | Moderate false positive rate |
Resource Intensive | Requires significant data processing | Less data-driven | Less resource-intensive |
Implementation Complexity | High; needs technical expertise | Lower; more straightforward | Moderate; easier than AI but complex rules may arise |
Analysis and Recommendations
AI-driven fraud detection presents a significant advantage in speed and adaptability compared to traditional and rule-based methods. Its ability to learn from vast amounts of data allows organizations to identify fraudulent activities quickly and with greater accuracy. While traditional methods may be easier to implement, they often lag in effectiveness against sophisticated cyber threats. Rule-based systems can provide a middle ground but may struggle to keep up with evolving fraud tactics.
Organizations should consider their specific needs, resources, and threat landscapes when choosing a fraud detection approach. For those with the capability to invest in technology and data processing, AI-driven solutions are highly recommended for robust and agile fraud detection. Conversely, smaller organizations with limited resources may find that traditional or rule-based systems better suit their immediate needs, albeit with the understanding that these methods may require eventual upgrades as threats evolve.
The Benefits of AI-Driven Fraud Detection and Software Management
-
Enhanced Security:
By leveraging AI technologies, organizations can significantly bolster their security measures. AI systems continuously monitor and analyze user behavior, enabling real-time detection of fraudulent activities. For example, if a user's behavior suddenly deviates from established patterns—such as logging in from an unusual location—AI can flag this activity for further investigation, thus protecting sensitive data.
-
Increased Efficiency:
AI automates many aspects of fraud detection and software management, reducing the burden on human resources. This means that teams can focus more on strategic initiatives rather than getting bogged down in manual monitoring and analysis. For instance, automated systems can quickly process vast amounts of data, identifying potential threats faster than traditional methods.
-
Cost Savings:
Implementing AI solutions can lead to substantial cost reductions over time. By preventing fraud before it occurs, organizations save significant amounts that would otherwise be lost. Additionally, the efficiency gained from automation can reduce operational costs associated with fraud investigations and software management.
-
Scalability:
As businesses grow, so do their data and transaction volumes. AI-driven systems can easily scale to handle increased loads without sacrificing performance. This adaptability ensures that organizations remain protected as they expand, allowing them to confidently pursue growth opportunities.
-
Data-Driven Insights:
AI not only protects organizations but also provides valuable insights into user behavior and trends. By analyzing data patterns, companies can gain a deeper understanding of their customer base, enabling them to make informed decisions that enhance user experience and drive engagement.
In summary, the integration of AI in fraud detection and software management offers a multitude of benefits, from enhanced security and efficiency to cost savings and scalability. These advantages empower organizations to stay ahead of cyber threats while also optimizing their operations, ultimately leading to a more secure and profitable business environment.
Use Cases for AI-Driven Fraud Detection
AI-driven solutions for fraud detection have found applications across various industries, showcasing their effectiveness in real-time risk mitigation. Below are some compelling use cases that illustrate the practical applications of these technologies.
Financial Services
In the financial sector, banks leverage machine learning algorithms to monitor transactions in real-time. For instance, a leading bank implemented an AI-based system that analyzes transaction patterns and identifies suspicious activities. As a result, they reduced fraudulent transactions by 30% within the first year of implementation, significantly enhancing customer trust and safeguarding financial assets.
E-Commerce
E-commerce platforms are increasingly adopting AI tools to detect fraudulent activities, such as account takeovers and false transactions. One major online retailer integrated an AI-driven fraud detection system that reviews user behaviors and flags anomalies. This proactive approach led to a 40% decrease in chargeback claims and improved overall customer satisfaction by ensuring a safer shopping environment.
Insurance
The insurance industry utilizes AI to streamline claims processing and detect fraudulent claims. A prominent insurance company employed an AI model that analyzes claim data in real-time, identifying patterns indicative of fraud. This initiative resulted in a 25% reduction in fraudulent claims, saving the company millions of dollars and allowing legitimate claims to be processed more efficiently.
Telecommunications
Telecommunications providers face significant challenges from subscription fraud. An innovative telecom company adopted an AI-driven solution to monitor new subscriber applications and flag potentially fraudulent accounts based on behavioral anomalies. This strategy led to a 50% reduction in subscription fraud and improved the accuracy of their customer onboarding process.
These use cases exemplify how AI-driven fraud detection solutions not only protect valuable assets but also enhance operational integrity across various sectors.
Conclusion and Call to Action
The integration of AI-driven solutions in fraud detection and software management is paving the way for more secure and efficient operational practices. Key findings reveal that machine learning algorithms not only enhance the accuracy of identifying fraudulent activities but also streamline response times, allowing organizations to act decisively in protecting their assets.
Looking ahead, we can anticipate a future where real-time analytics and AI capabilities become standard in cybersecurity frameworks. As cyber threats continue to evolve, the ability to swiftly adapt and respond using intelligent technologies will be paramount for maintaining operational integrity and safety.
To stay ahead of the curve in this rapidly changing landscape, we encourage you to explore additional resources on AI-driven fraud detection strategies. Consider joining our community of experts and practitioners, or reach out to our team for tailored insights and solutions that can help your organization navigate these challenges effectively.
Contact Us
Tell Us About Your Project
Shoot Us a Call
Get ahold of our consultation team today to discuss your development needs!
Email Us
If you'd prefer to message us directly, no problem! We will respond as soon as possible.